Microsoft’s most capable new Phi 4 AI model rivals the performance of far larger systems
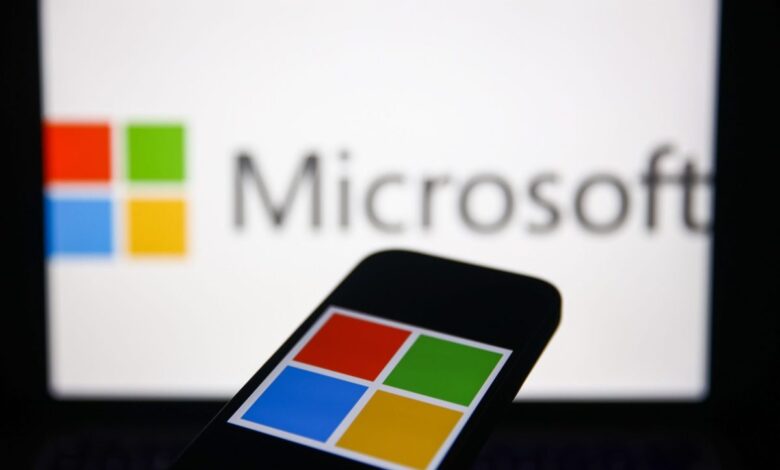
Microsoft launched several new “open” AI models on Wednesday, the most capable of which is competitive with OpenAI’s o3-mini on at least one benchmark.
All of the new pemissively licensed models — Phi 4 mini reasoning, Phi 4 reasoning, and Phi 4 reasoning plus — are “reasoning” models, meaning they’re able to spend more time fact-checking solutions to complex problems. They expand Microsoft’s Phi “small model” family, which the company launched a year ago to offer a foundation for AI developers building apps at the edge.
Phi 4 mini reasoning was trained on roughly 1 million synthetic math problems generated by Chinese AI startup DeepSeek’s R1 reasoning model. Around 3.8 billion parameters in size, Phi 4 mini reasoning is designed for educational applications, Microsoft says, like “embedded tutoring” on lightweight devices.
Parameters roughly correspond to a model’s problem-solving skills, and models with more parameters generally perform better than those with fewer parameters.
Phi 4 reasoning, a 14-billion-parameter model, was trained using “high-quality” web data as well as “curated demonstrations” from OpenAI’s aforementioned o3-mini. It’s best for math, science, and coding applications, according to Microsoft.
As for Phi 4 reasoning plus, it’s Microsoft’s previously-released Phi-4 model adapted into a reasoning model to achieve better accuracy on particular tasks. Microsoft claims that Phi 4 reasoning plus approaches the performance levels of R1, a model with significantly more parameters (671 billion). The company’s internal benchmarking also has Phi 4 reasoning plus matching o3-mini on OmniMath, a math skills test.
Phi 4 mini reasoning, Phi 4 reasoning, and Phi 4 reasoning plus are available on the AI dev platform Hugging Face accompanied by detailed technical reports.
Techcrunch event
Berkeley, CA
|
June 5
“Using distillation, reinforcement learning, and high-quality data, these [new] models balance size and performance,” wrote Microsoft in a blog post. “They are small enough for low-latency environments yet maintain strong reasoning capabilities that rival much bigger models. This blend allows even resource-limited devices to perform complex reasoning tasks efficiently.”